Xuan Cao; Junfang Jiang, Baidu
One of the most important works for SREs is troubleshooting the problem causing KPI degradation such as decrease of PV, advertisement income, feed click rate, etc.
Many of the problems only affect a portion of the incoming traffic. If the on-call engineers can learn about the characteristics of the affected portion, such as one of traffic source area, browser type or access network standard, then diagnosis would be accelerate.
Therefore, we mark a set of tags on each user request. When a failure happens, we look for the common points among the faulty requests. This generates a huge amount of tagged data, which increase the searching scope and thus leading to low efficiency in trouble shooting, therefore automatic analysis is imperative.
In this talk, we will present our work in Baidu where we apply machine learning techniques to recommend the tags most relevant to the failure. This approach adopts unsupervised anomaly detection and entropy-based dimension reduction techniques, which can automatically recommend key data features for troubleshooting. The proposed approach has been validated by hundreds of real cases. It significantly speeds up the troubleshooting procedure when compared to traditional approaches.
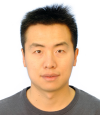
Open Access Media
USENIX is committed to Open Access to the research presented at our events. Papers and proceedings are freely available to everyone once the event begins. Any video, audio, and/or slides that are posted after the event are also free and open to everyone. Support USENIX and our commitment to Open Access.

author = {Xuan Cao and Junfang Jiang},
title = {Efficient Trouble Shooting of Service Failures with {Multi-Tag} Data Analysis},
year = {2018},
publisher = {USENIX Association},
month = jun
}