Patricia Thaine, Private AI, University of Toronto; Pieter Luitjens, Private AI; Dr. Parinaz Sobhani, Georgian Partners
This talk is a guide to using privacy technology in deployment. First, we will give a brief overview of the current state of privacy technology for (a) Differential Privacy & Anonymization, and (b) Secure Multiparty Computation, Homomorphic Encryption, Secure Enclaves. We will then go over the current obstacles of deploying privacy-preserving software; namely, identifying privacy risks & risk management, the capabilities & limitations of privacy tool sets and the backgrounds required to use them. Obstacles differ depending on whether one is attempting to retrofit a codebase in order to integrate privacy post-hoc or whether one is choosing the tech stack they will use for creating a codebase that integrates Privacy by Design. With those two scenarios in mind, we will discuss strategies for choosing privacy tools, for choosing to compute on the edge vs. on-premise vs. on the cloud, and for thinking about right risk management frameworks.
Patricia Thaine, Private AI, University of Toronto
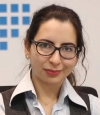
Pieter Luitjens, Private AI

Parinaz Sobhani, Georgian Partners


author = {Patricia Thaine and Pieter Luitjens and Parinaz Sobhani},
title = {Privacy in Deployment},
booktitle = {2020 {USENIX} Conference on Privacy Engineering Practice and Respect ({PEPR} 20)},
year = {2020},
url = {https://www.usenix.org/conference/pepr20/presentation/thaine},
publisher = {USENIX Association},
month = oct
}